Parametric Inference and Process Control: A Comprehensive Guide for Advanced Statistical Analysis

In today's data-driven world, statistical inference plays a pivotal role in making informed decisions. Parametric inference, a powerful statistical technique, provides a robust framework for estimating and testing hypotheses about unknown population parameters. This article delves into the intricacies of parametric inference and its indispensable applications in process control, offering a comprehensive guide for readers seeking to master these advanced statistical concepts.
Parametric inference revolves around the assumption that the data under investigation follows a specific probability distribution, known as a parametric distribution. This assumption allows researchers to make inferences about the population parameters, such as the mean, standard deviation, or proportion, based on a sample of data.
The key to parametric inference lies in the selection of an appropriate probability distribution that best fits the data. Common probability distributions include the normal distribution, the binomial distribution, and the exponential distribution, each representing a different type of data distribution.
5 out of 5
Language | : | English |
File size | : | 4665 KB |
Print length | : | 147 pages |
Maximum likelihood estimation (MLE) is a fundamental technique in parametric inference used to estimate the unknown population parameters. MLE involves finding the values of the parameters that maximize the likelihood function, a measure of the probability of observing the data given the assumed distribution.
By solving the likelihood equations, researchers can obtain point estimates of the population parameters, providing a best guess for the true values. MLE plays a crucial role in hypothesis testing, forming the basis for constructing confidence intervals and conducting significance tests.
Hypothesis testing is a cornerstone of statistical inference, enabling researchers to draw s about population parameters based on sample data. A hypothesis test starts with formulating null and alternative hypotheses that represent different claims about the population parameters.
Using the MLE estimates and the sampling distribution of the test statistic, researchers can calculate the p-value, which represents the probability of observing the sample data or more extreme values under the null hypothesis. If the p-value is sufficiently low, the null hypothesis is rejected, suggesting that the alternative hypothesis is more plausible.
Parametric inference finds extensive applications in process control, where it empowers engineers and quality control professionals to monitor and improve industrial processes. By modeling process data using parametric distributions, such as the normal distribution or the Weibull distribution, they can:
- Estimate process parameters: Calculate key characteristics of the process, such as the average output, variation, and defect rate.
- Establish control limits: Determine acceptable ranges for process variables based on the estimated parameters, ensuring that the process operates within desired specifications.
- Detect process shifts: Monitor the process over time and detect deviations from the expected parameters, indicating potential problems or areas for improvement.
- Optimize process performance: Use statistical techniques, such as design of experiments, to identify optimal process settings and improve efficiency and quality.
Parametric inference has revolutionized industries by providing valuable insights into complex data. Consider these real-world examples:
- Manufacturing: In a semiconductor manufacturing plant, parametric inference is used to estimate the mean and standard deviation of wafer thickness. By setting control limits based on these parameters, engineers can identify and correct process variations, improving wafer yield and reducing defects.
- Healthcare: In a clinical trial, parametric inference is employed to compare the effectiveness of two treatments. By estimating the mean treatment effect and conducting hypothesis tests, researchers can determine whether one treatment is statistically superior to the other.
- Finance: In financial modeling, parametric inference is used to estimate the parameters of probability distributions that describe stock prices or interest rates. This information is vital for risk assessment, portfolio optimization, and forecasting financial trends.
Parametric inference is a powerful tool that empowers researchers, engineers, and professionals to make informed decisions based on data. By assuming a specific probability distribution and utilizing techniques like MLE and hypothesis testing, parametric inference enables the estimation and testing of population parameters, uncovering hidden insights and driving process improvements.
Whether you are a statistician, a process engineer, or simply curious about the art of statistical inference, Parametric Inference and Process Control: Springerbriefs in Statistics offers a comprehensive guide to this essential statistical technique. Delve into the pages of this book to master the theory, applications, and real-world examples of parametric inference, and unlock the power of data analysis for your own research and professional endeavors.
5 out of 5
Language | : | English |
File size | : | 4665 KB |
Print length | : | 147 pages |
Do you want to contribute by writing guest posts on this blog?
Please contact us and send us a resume of previous articles that you have written.
Book
Novel
Page
Chapter
Text
Story
Genre
Reader
Library
Paperback
E-book
Magazine
Newspaper
Paragraph
Sentence
Bookmark
Shelf
Glossary
Bibliography
Foreword
Preface
Synopsis
Annotation
Footnote
Manuscript
Scroll
Codex
Tome
Bestseller
Classics
Library card
Narrative
Biography
Autobiography
Memoir
Reference
Encyclopedia
Paul K Saint Amour
Stanley Rachman
John Allan
Najeeb Shaikh
K E C
Sandeep Koranne
David Presnell
Alberto Caballero
Jillian Grose Fifer
H Dale Burke
True Crime Seven
Iain Gordon
Amit Chilka
Clarain Marvellio
George Papadopoulos
Tom Shepherd
James L Garlow
Tanya Simons
Souvankham Thammavongsa
Lesslie Newbigin
Light bulbAdvertise smarter! Our strategic ad space ensures maximum exposure. Reserve your spot today!
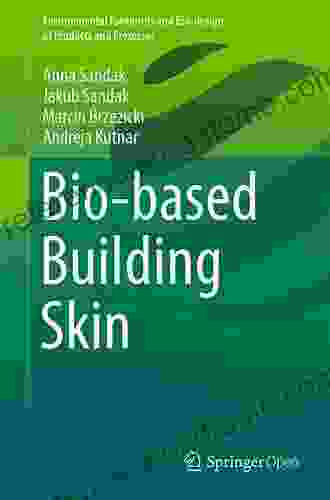

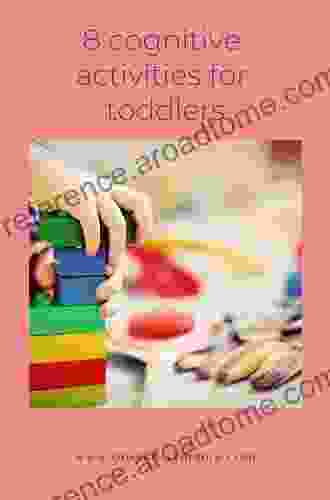

- Cason CoxFollow ·5.1k
- Mark TwainFollow ·3k
- Damon HayesFollow ·7.5k
- Howard BlairFollow ·2.8k
- Desmond FosterFollow ·19.5k
- Luke BlairFollow ·9.6k
- Mikhail BulgakovFollow ·10.2k
- Harold PowellFollow ·17k
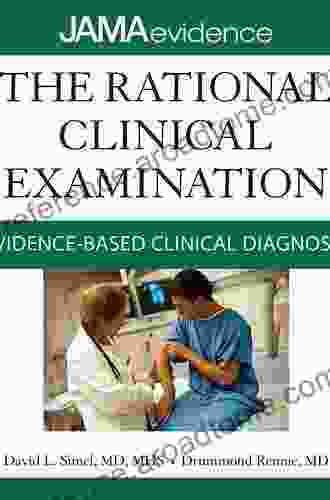

Unlock the Secrets of Accurate Clinical Diagnosis:...
Harnessing the Power of...
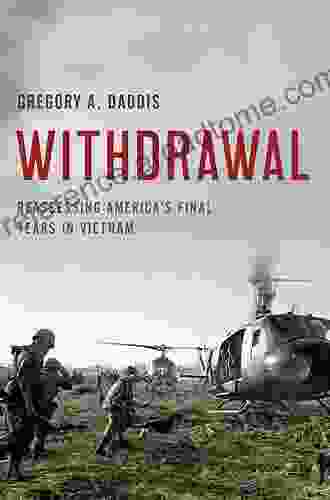

Withdrawal: Reassessing America's Final Years in Vietnam
The Controversial...
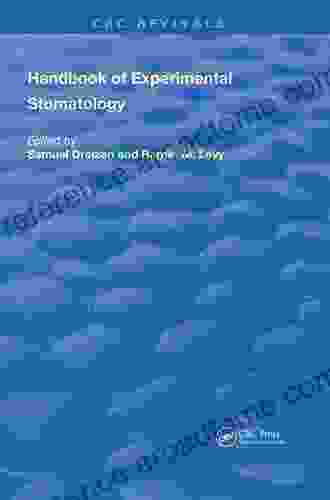

Handbook Of Experimental Stomatology: Routledge Revivals
About the Book The...
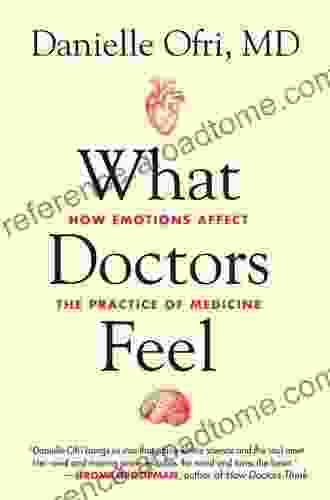

Unveiling the Profound Impact of Emotions on Medical...
In the realm of healthcare, the focus has...
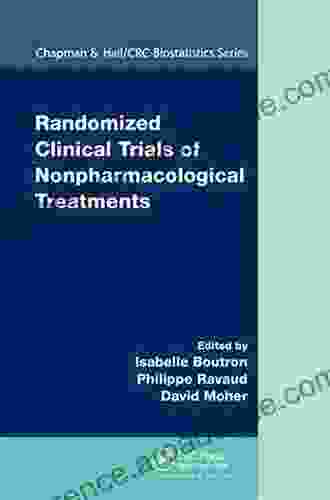

Randomized Clinical Trials of Nonpharmacological...
In the ever-evolving field of...
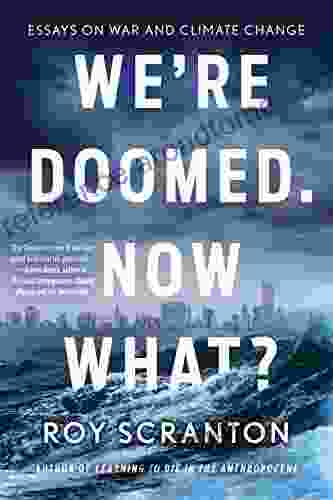

Essays on War and Climate Change: A Literary Examination...
In an era marked by...
5 out of 5
Language | : | English |
File size | : | 4665 KB |
Print length | : | 147 pages |