Marginal Models in Analysis of Correlated Binary Data with Time Dependent

Binary data, where outcomes can only take two possible values (0 or 1),is commonly encountered in research across various fields. When analyzing binary data, it is important to consider potential correlations between observations, especially when data is collected over time. Marginal models provide a powerful approach to handle such correlated binary data, allowing researchers to model the probability of an event occurring while accounting for time-dependent effects.
5 out of 5
Language | : | English |
File size | : | 7116 KB |
Screen Reader | : | Supported |
Print length | : | 189 pages |
X-Ray for textbooks | : | Enabled |
Marginal Models for Correlated Binary Data
Marginal models are a class of generalized linear models that are specifically designed for analyzing correlated binary data. They allow researchers to model the marginal probability of an event occurring for each individual observation, while accounting for the correlation between observations. Marginal models are particularly useful when the correlation structure is complex or unknown, as they do not require the specification of a specific correlation structure.
Model Formulation
A marginal model for correlated binary data can be formulated as follows:
$$ logit[P(Y_i = 1 | X_i, Z_i)] = \beta_0 + \beta_1 X_i + f(Z_i) $$
- $Y_i$ is the binary outcome variable
- $X_i$ is a vector of independent variables
- $Z_i$ is a vector of time-dependent variables
- $\beta_0$ is the intercept
- $\beta_1$ is a vector of regression coefficients
- $f(.)$ is a smooth function that models the time-dependent effects
The smooth function $f(.)$ can take various forms, such as a linear function, a polynomial function, or a nonparametric function. The choice of the smooth function depends on the nature of the time-dependent effects.
Parameter Estimation
The parameters of a marginal model can be estimated using maximum likelihood estimation. The likelihood function for a marginal model is given by:
$$ L(\beta, \phi) = \prod_{i=1}^n [P(Y_i = 1 | X_i, Z_i)]^{Y_i}[1 - P(Y_i = 1 | X_i, Z_i)]^{1 - Y_i}$$
- $\beta$ is the vector of regression coefficients
- $\phi$ is the vector of parameters of the smooth function $f(.)$
The maximum likelihood estimates of $\beta$ and $\phi$ can be obtained by maximizing the likelihood function using iterative algorithms, such as the Fisher scoring algorithm.
Interpretation
The interpretation of marginal models is similar to the interpretation of logistic regression models. The regression coefficients $\beta_1$ represent the effect of the independent variables on the log-odds of the event occurring. The smooth function $f(.)$ captures the time-dependent effects on the log-odds of the event occurring. The slope of the smooth function indicates the rate of change of the log-odds over time.
Applications
Marginal models have a wide range of applications in various fields, including:
- Epidemiology: Analysis of longitudinal binary outcomes, such as disease incidence or recovery
- Medical research: Analysis of binary outcomes in clinical trials with repeated measurements
- Social science: Analysis of binary outcomes in longitudinal surveys
- Economics: Analysis of binary outcomes in panel data
Marginal models provide a powerful approach to analyze correlated binary data with time-dependent effects. They allow researchers to model the marginal probability of an event occurring while accounting for the correlation between observations, and to identify the effects of time-dependent variables on the outcome. Marginal models are a valuable tool for researchers working with binary data, and they can provide meaningful insights into the relationships between variables in a wide range of applications.
References
- Fahrmeir, L., Tutz, G., & Hennevogl, W. (2004). Multivariate statistical modelling using generalized linear models. Springer Science & Business Media.
- McCulloch, C. E., & Nelder, J. A. (2019). Generalized linear models. CRC Press.
- Diggle, P. J., Heagerty, P., Liang, K. Y., & Zeger, S. L. (2002). Analysis of longitudinal data. Oxford University Press.
5 out of 5
Language | : | English |
File size | : | 7116 KB |
Screen Reader | : | Supported |
Print length | : | 189 pages |
X-Ray for textbooks | : | Enabled |
Do you want to contribute by writing guest posts on this blog?
Please contact us and send us a resume of previous articles that you have written.
Book
Novel
Page
Chapter
Text
Story
Genre
Reader
Library
Paperback
E-book
Magazine
Newspaper
Paragraph
Sentence
Bookmark
Shelf
Glossary
Bibliography
Foreword
Preface
Synopsis
Annotation
Footnote
Manuscript
Scroll
Codex
Tome
Bestseller
Classics
Library card
Narrative
Biography
Autobiography
Memoir
Reference
Encyclopedia
Conrad V Christensen
Thomas Brandt
Peter Viereck
Efrat Haddi
Scott Atran
Trang Nguyen
Karen Sullivan
6th Revised Edition Kindle Edition
Matthew R Kutz
Benjamin Lai
Edred Thorsson
Amanda Evans
Alberto Caballero
Sohrab Ahmari
Michele A Livingston
Georg Feuerstein
Jerramy Fine
Mary Sue Englund
Seyyed Hossein Nasr
1st Ed 2020 Edition
Light bulbAdvertise smarter! Our strategic ad space ensures maximum exposure. Reserve your spot today!
- Duane KellyFollow ·16.8k
- Ralph Waldo EmersonFollow ·18.6k
- Giovanni MitchellFollow ·19.5k
- Lawrence BellFollow ·5.9k
- Mitch FosterFollow ·13.8k
- Gene PowellFollow ·8.9k
- Junichiro TanizakiFollow ·14k
- Mario BenedettiFollow ·8.6k
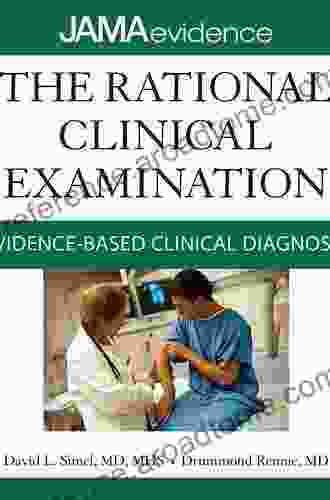

Unlock the Secrets of Accurate Clinical Diagnosis:...
Harnessing the Power of...
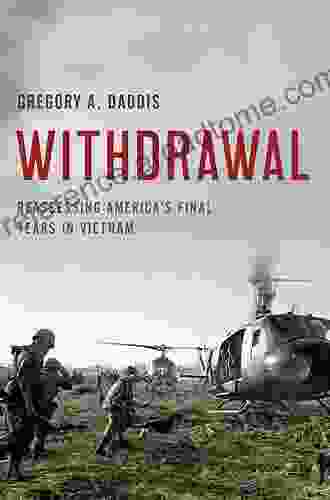

Withdrawal: Reassessing America's Final Years in Vietnam
The Controversial...
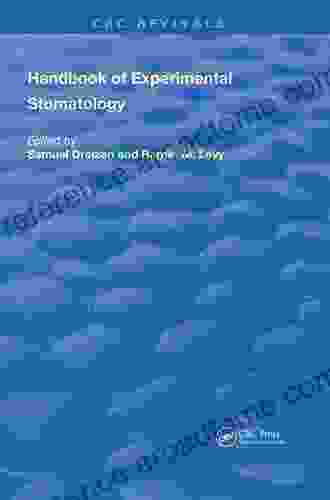

Handbook Of Experimental Stomatology: Routledge Revivals
About the Book The...
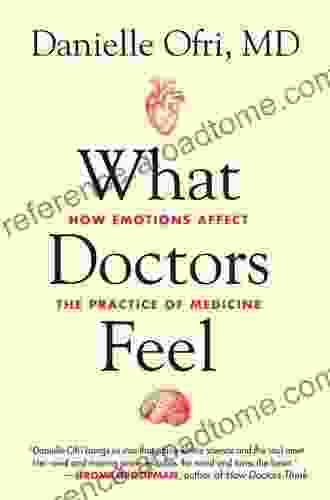

Unveiling the Profound Impact of Emotions on Medical...
In the realm of healthcare, the focus has...
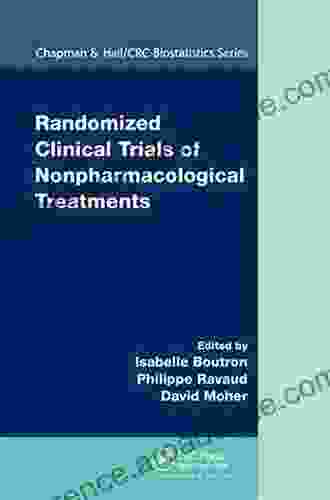

Randomized Clinical Trials of Nonpharmacological...
In the ever-evolving field of...
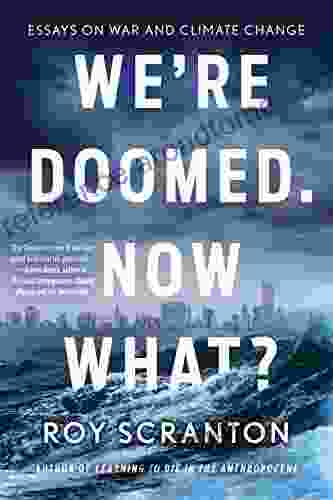

Essays on War and Climate Change: A Literary Examination...
In an era marked by...
5 out of 5
Language | : | English |
File size | : | 7116 KB |
Screen Reader | : | Supported |
Print length | : | 189 pages |
X-Ray for textbooks | : | Enabled |