Predictive Models for Decision Support in the COVID-19 Crisis: Empowering Healthcare Systems and Policymakers

The unprecedented COVID-19 pandemic has posed unprecedented challenges to healthcare systems and policymakers worldwide. The rapid spread of the virus and its unpredictable nature demanded swift and informed decision-making at every level. Predictive models emerged as a critical tool in this crisis, providing valuable insights into the virus's transmission, potential spread, and the impact of various interventions.
Predictive models are mathematical frameworks that utilize data to forecast future events or outcomes. In the context of the COVID-19 crisis, predictive models played a crucial role in:
Projecting the Spread of the Virus: Models estimated the likely transmission rate, reproduction number, and potential spread of the virus in different regions and populations. This information guided containment measures, social distancing guidelines, and resource allocation.
Predictive Models for Decision Support in the COVID-19 Crisis (SpringerBriefs in Applied Sciences and Technology)by Turgon Annárë4.2 out of 5
Language : English File size : 27169 KB Text-to-Speech : Enabled Screen Reader : Supported Enhanced typesetting : Enabled Word Wise : Enabled Print length : 147 pages Forecasting Healthcare Demand: Models predicted the surge in demand for healthcare resources, including hospital beds, ventilators, and medical personnel. These forecasts helped healthcare systems prepare and optimize their capacity, preventing potential shortages and ensuring adequate care for patients.
Evaluating Intervention Strategies: Models assessed the effectiveness of various interventions, such as lockdowns, travel restrictions, and vaccination campaigns. They quantified the impact of these measures on virus transmission, healthcare demand, and economic activity, informing policy decisions and prioritizing resources.
Identifying Vulnerable Populations: Models identified populations at high risk of severe illness or death from COVID-19 based on factors such as age, underlying health conditions, and socioeconomic status. This information guided targeted protection measures and tailored healthcare interventions.
Numerous types of predictive models were employed to address the complex challenges posed by the COVID-19 pandemic, including:
Epidemiological Models: These models focused on simulating the transmission of the virus and predicting the course of the outbreak. They incorporated factors such as population density, contact rates, and immunity levels.
Machine Learning Models: These models utilized advanced algorithms to identify patterns and relationships in data. They were used to predict individual risk of infection, estimate the prevalence of asymptomatic cases, and forecast healthcare demand.
Integrated Models: These models combined different types of models to provide a comprehensive view of the pandemic. They incorporated epidemiological data, population demographics, and economic factors to generate more accurate and robust forecasts.
Developing and using predictive models in the COVID-19 crisis presented several challenges:
Data Availability and Quality: The availability and quality of data were critical for accurate predictions. Collecting reliable data on infection rates, hospitalizations, and deaths was essential, but often challenging due to limited testing, reporting inconsistencies, and data delays.
Model Complexity and Assumptions: Predictive models can be complex and rely on numerous assumptions. Balancing model complexity with interpretability was crucial to ensure that results were reliable and accessible to decision-makers.
Uncertainty and Model Validation: The rapidly evolving nature of the pandemic and the incomplete understanding of the virus introduced significant uncertainty into predictive models. Validating models and assessing their accuracy was essential to build confidence in their predictions.
Despite the challenges, predictive models played a significant role in informing decision-making in numerous countries during the COVID-19 crisis:
In the United Kingdom, the Health Protection Surveillance Centre (HPSC) used predictive models to project hospital bed demand, optimize ventilator allocation, and guide lockdown measures. These models helped the healthcare system prepare for the surge in cases and mitigate the impact on the healthcare workforce.
In Singapore, the Ministry of Health (MOH) employed a range of predictive models to estimate the risk of importation and spread of COVID-19. These models informed bFree Download control measures, contact tracing efforts, and mass testing strategies, resulting in one of the lowest COVID-19 mortality rates globally.
In the United States, the Centers for Disease Control and Prevention (CDC) utilized predictive models to forecast the potential impact of COVID-19 on healthcare systems and populations. These models guided national guidelines, resource allocation, and vaccine distribution, helping mitigate the severity of the outbreak.
Predictive models emerged as a powerful tool in the fight against the COVID-19 pandemic, providing valuable insights into the virus's transmission, healthcare demand, and the impact of interventions. By leveraging data and advanced algorithms, predictive models empowered healthcare systems and policymakers to make informed decisions, optimize resource allocation, and save lives. As the world continues to navigate the ongoing pandemic and future health crises, predictive models will remain a critical asset in informing decision-making and ensuring preparedness.
- Image 1: A visualization of a predictive model simulating the spread of COVID-19 through a population network.
- Image 2: A graph depicting the predicted healthcare demand for a specific region, based on a predictive model.
- Image 3: A dashboard displaying the results of a predictive model, providing real-time insights into the COVID-19 outbreak.
- Image 4: A global map showing the predicted risk of COVID-19 importation and spread, informed by predictive models.
- Image 5: A group of experts using predictive models to analyze data and inform decision-making during the COVID-19 pandemic.
4.2 out of 5
Language | : | English |
File size | : | 27169 KB |
Text-to-Speech | : | Enabled |
Screen Reader | : | Supported |
Enhanced typesetting | : | Enabled |
Word Wise | : | Enabled |
Print length | : | 147 pages |
Do you want to contribute by writing guest posts on this blog?
Please contact us and send us a resume of previous articles that you have written.
Book
Novel
Page
Chapter
Text
Story
Genre
Reader
Library
Paperback
E-book
Magazine
Newspaper
Paragraph
Sentence
Bookmark
Shelf
Glossary
Bibliography
Foreword
Preface
Synopsis
Annotation
Footnote
Manuscript
Scroll
Codex
Tome
Bestseller
Classics
Library card
Narrative
Biography
Autobiography
Memoir
Reference
Encyclopedia
Eve Bunting
Bruce Tate
Robin Randall
Jinghao Zhou
Iva Lloyd
Dean Wampler
Benjamin B Cohen
Charles P Lutz
Esther W
Aep Saepudin
Mike Gibney
Karen Adler
Stephen Ball
Laura Berman Fortgang
32nd Edition Kindle Edition
Jane King
Anna De Fina
Angelo Luongo
Stefanie Syman
David Levine Phd Pt
Light bulbAdvertise smarter! Our strategic ad space ensures maximum exposure. Reserve your spot today!
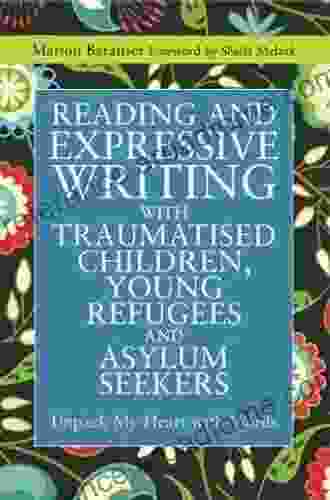

- Oscar WildeFollow ·6.6k
- Norman ButlerFollow ·17.6k
- Floyd PowellFollow ·9.2k
- Desmond FosterFollow ·19.5k
- Jeffery BellFollow ·16k
- Henry JamesFollow ·19.6k
- Jett PowellFollow ·15.8k
- Allen ParkerFollow ·14.1k
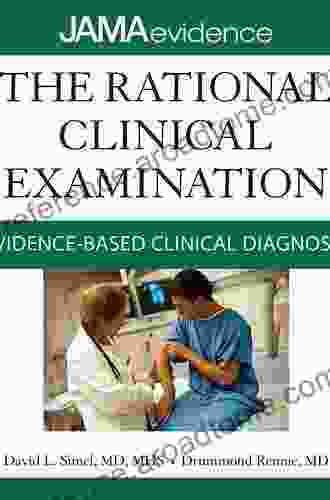

Unlock the Secrets of Accurate Clinical Diagnosis:...
Harnessing the Power of...
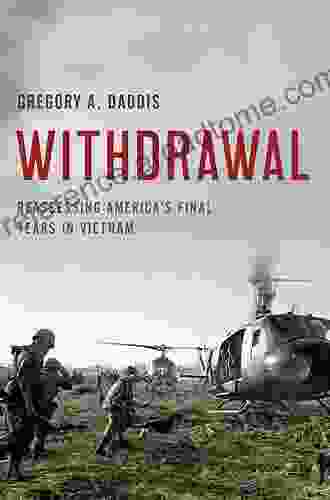

Withdrawal: Reassessing America's Final Years in Vietnam
The Controversial...
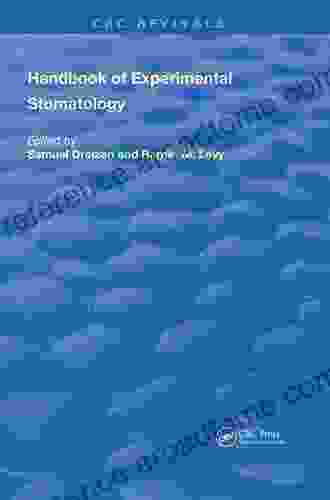

Handbook Of Experimental Stomatology: Routledge Revivals
About the Book The...
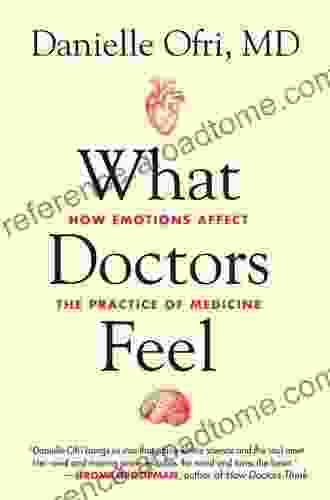

Unveiling the Profound Impact of Emotions on Medical...
In the realm of healthcare, the focus has...
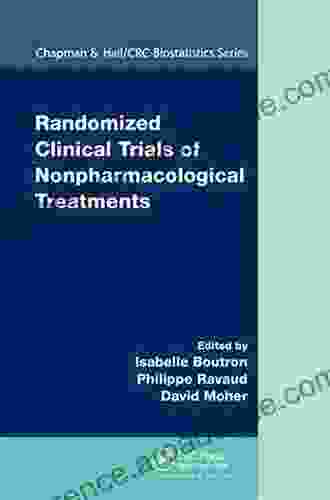

Randomized Clinical Trials of Nonpharmacological...
In the ever-evolving field of...
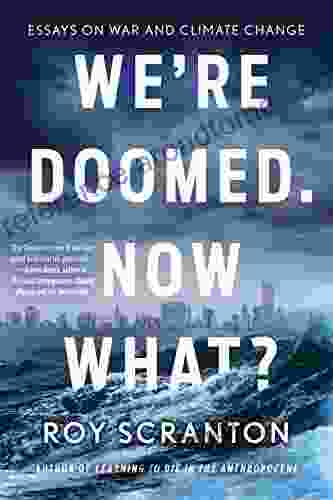

Essays on War and Climate Change: A Literary Examination...
In an era marked by...
4.2 out of 5
Language | : | English |
File size | : | 27169 KB |
Text-to-Speech | : | Enabled |
Screen Reader | : | Supported |
Enhanced typesetting | : | Enabled |
Word Wise | : | Enabled |
Print length | : | 147 pages |